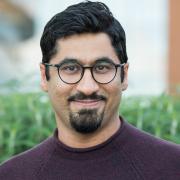
Reza Abbasi-Asl, PhD
Research description
Our lab investigates the role of advanced computational tools in understanding brain functions and its related disorders. More specifically, we develop interpretable machine learning tools to (1) integrate multi-modal data collected from the brain (and body) in both microscopic and macroscopic resolutions, (2) predict functions of biological systems in different resolutions, and (3) determine the functional differences across neurological disorders. We are a part of the Neuroscape Center and Bakar Computational Health Sciences Institute at UCSF and our team is supported through funding from NIH National Institute of Mental Health, NIH National Institute of Aging, Weill Neurohub, Sandler Program for Breakthrough in Biomedical Research, UCSF Innovation Ventures, and Google.
Current projects
Understanding the relationship between neural function and connectivity in mouse visual cortex: Characterizing the relationship between neural function and connectivity is a central problem in visual sensory processing. To explore this relationship, we study visual responses from neurons within an 800X800 um region of primary visual cortex in adult mouse, spanning all visual layers from pia to white matter. The data was collected at the Allen Institute and includes ~80,000 neurons in 4 mice, collected from 750 2-photon and 35 3-photon calcium imaging planes. Enabled by a BRAIN Initiative RF1 award, our research lab has been developing tools to analyze this dataset and examine the single-cell and population activity in primary visual cortex in response to a wide variety of stimuli. We also explore the relationship between neural responses and neural morphology/connectivity captured by a large-scale electron microscopy dataset from the same tissue.
Development of biologically realistic models of neurons enabled by interpretable machine learning: We have worked alongside the modeling team at Allen Institute on biophysically detailed models of neurons in mouse cortex. Our previous studies outline details of this bio-realistic model for primary visual cortex in mouse. This model benefits from our recent understanding of diverse cell-types in cortex. In an ongoing collaboration with the Allen Institute, we are leading an effort to establish highly predictive combined bio-realistic and neural network models for cortex. These models benefit from accurate artificial neural network module in their structure. Combined with the bio-realistic module, these hybrid models can accurately predict neural responses and characterize the role of diverse cell-types in the visual cortex.
Unsupervised pattern discovery in whole-brain spatial transcriptomics: The growth of large-scale spatial gene expression data requires new computational tools to extract major trends in gene expression in their native spatial context. We have previously developed unsupervised and interpretable computational frameworks to identify spatial segments and principal patterns in brain-wide gene expression datasets. These frameworks are based on interpretable and stability-driven machine learning principles. Using these frameworks, our lab has discovered stable and spatially coherent regions of the mouse brain, potentially without human labor, error, and bias. These regions offer new brain patterns that are highly correlated with combinations of expert-annotated brain regions, while also reaching beyond the established mouse brain ontology. Our ongoing projects are focused on the development of similar frameworks for brain-wide spatial transcriptomics datasets in mouse and human including MERFISH datasets collected under the Allen Brain Cell Atlas.
Neural correlates of motor and cognitive decline in neurological disorders captured by DBS implants, multi-modal bio-sensing, and wearables: Multi-modal biosensor and imaging systems in precision health have emerged as an effective tool to capture disease symptoms and progression. With the recent developments in high-resolution acquisition of multimodal physiological data from the human brain and body, there is an emerging need to design specialized computational systems that determine neural correlates of motor and cognitive decline. Our team is focused on the integration of multi-modal biosensing data to track progression in neurological diseases such as Multiple Sclerosis (MS), Parkinson’s disease (PD), and mood disorder. This includes development of machine learning models enabled by video/audio/biosensor/wearable recordings that accurately predict and track neurological symptoms in PD or MS and determine brain biomarkers of symptoms captured by neurophysiological measurements or high-resolution brain imaging.
Lab Members
Arefeh Sherafati
Postdoctoral scholar
Patrick Xian
Postdoctoral Scholar
Maryam Bijanzadeh
Assistant Professional Researcher, Neuroscape
Gavin Cui
PhD Candidate
Alex Lee
PhD Candidate
Russell Ro
PhD Student
Kara Presbrey
PhD Candidate
Maria Olaru
PhD Candidate
Max Collard
MD/PhD Student
Lab Website
Academic community service and committee membership: Director of the Data Analytics at the UCSF Weill Institute for Neuroscience. Executive Committee member at the UCSF Bakar Computational Health Sciences Institute. BMI and BioE program admission committees. UCSF RAP program's Permanent Reviewer. UCSF BBC Seminar Committee. Weill Neurosciences Building Server Room Committee. Neuroscape Center Staff Advancement and Promotion Committee. TRAIN UP: Inclusive Research Mentor Course - Supervising, Training, and Advising Training and UCSF Coro Faculty Leadership Training. Former Chair of the Educational Committee at the IEEE Brian Initiative.